Nothing in biology makes sense
except properly quantified in the light of evolution.
Evolutionary systems biology is ultimately about rigorously quantifying the evolution of biological systems. To achieve this, EvoSysBio combines the rigor of population genetics with approaches from current systems biology to formulate testable hypotheses (see Figure 1). Discussions over a series of workshops have substantially contributed to shaping the following definition of EvoSysBio (see EvoSysBio in 10 Slides):
Evolutionary Systems Biology
aims to ultimately generate realistic, testable,
integrated, dynamic, and interactive overviews
of multi-dimensional fitness landscapes
that mechanistically predict
① fitness changes caused by transitions
between potential states of individuals
and/or their environments, and
② evolutionary paths of changing
populations of such individuals
as they travel through state-space.
More on background, challenges, and reasons motivating this
rigorous definition of mechanistic EvoSysBio is available elsewhere (Loewe, 2016).
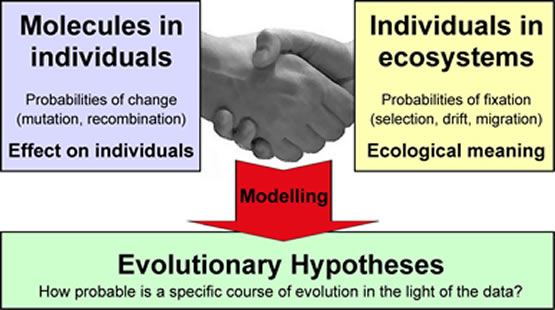
Figure 1: EvoSysBio combines the intra-individual approaches of current systems biology with the population level approaches of population genetics and ecology. From Loewe (2009); see Loewe (2016) for a version that defines clearer boundaries in terms of the Intra-Organism Biology and Trans-Organism Biology involved.
Modelling efforts in computational molecular systems biology provide unique opportunities to quantify how genotypes map to phenotypes. Such work can contribute much to quantifying the fitness landscape that governs the evolution of life. Unfortunately this important concept for evolutionary biology is poorly quantified for almost all biological systems and many discussions of fitness landscapes are at best heuristic (for exceptions, see e.g. Dean & Thornton 2007, Lande 2008 and other refs in Loewe 2009). This dearth of data and difficulties in visualizing their multi-dimentional trait-spaces conspire to render most known visualizations of fitness landscapes to become so-called "MOCA-LIFTs" (acronym for Massively Oversimplified Cartoons of Abstracted Landscapes of Incomplete Fitness Traits), like the following example:
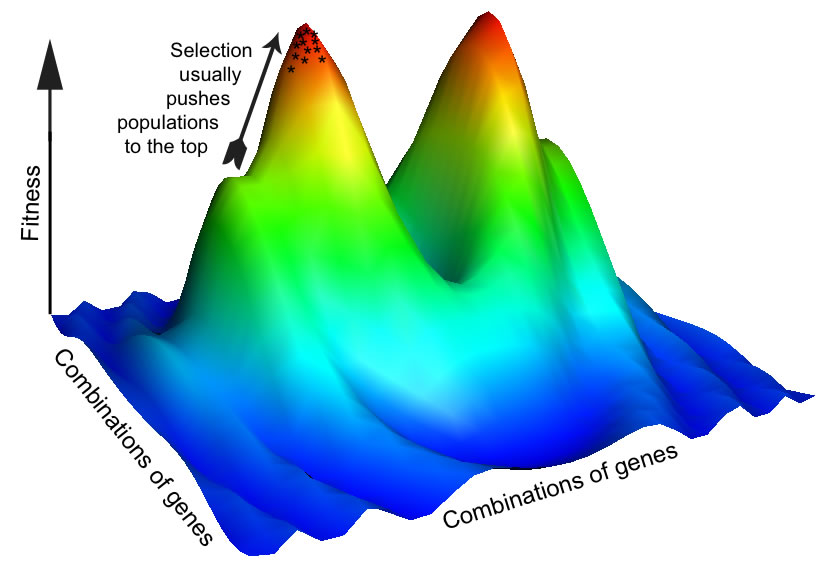
Figure 2: Exemplary MOCA-LIFT (Massively Oversimplified Cartoons of Abstracted Landscapes of Incomplete Fitness Traits).
If Incomplete Fitness Traits such as survival or fecundity increase the 'height' for a given lateral move in the plane, then it is easy to see why selection over many generations of varying offspring pushes most populations towards the next locally reachable optimum.
Each observable real-world Landscape of Incomplete Fitness Traits (LIFT) is in principle defined by a 'Height' that exists for every point in a 'Plane'. The Height is a more consequential Incomplete Fitness Trait (IFT) that is often multi-dimensional, but never actual fitness that is much more difficult to define. The relevant Plane represents one or more causal IFTs, as defined by biologically informed mechanistic simulations or by corresponding real-world measurements in the lab (e.g. of quantitative genetic traits, or combinations of genes). Computing or measuring this 'height' and navigating the 'plane' is extraordinary difficult for most biological systems. Thus, many discussions of fitness landscapes resort to the use of MOCA-LIFTs like this one. EvoSysBio aims to infuse more real-world data into such discussions.
The many levels of biological organization and interaction make it particularly difficult to quantify the adaptive landscape and both, 'plane' and 'height' routinely reflect high-dimensional spaces. To break this big problem down into more addressable ones, Loewe (2009) proposed that points that help quantify the adaptive landscape can be part of any of the following seven levels of the adaptive landscape.
- A molecular structure in the space of genotypes (addressed by structural biology, e.g. by protein structure prediction programs)
- A molecular function in the space of molecular structures (addressed by structural biology, comparative biology and biochemical experiments, e.g. by measuring kinetic rates or predicting them by interpolation between known rates of enzymes with known structures)
- A computable emergent property in the space of molecular functions (addressed by current systems biology via computer simulations of biochemical networks, e.g. by computing the flux through a pathway)
- Simulations of Incomplete Fitness Traits (SIFTs), which are computable emergent properties determined by the time series that result from the many interactions among diverse components within organisms (with a bit of biological expertise it is usually possible to identify which measures of time series impact high-level fitness traits like survival or fecundity)
- An Real-world observations of Incomplete Fitness Traits (RIFTs) will map well to their simulated counterparts (SIFTS) if indeed the system modeled has been understood completely and the right measures were picked for defining IFTs; otherwise it involves the construction of heuristic quantitative links, such as computing predictions of biomass growth that are tuned to be proportional observed growth rates of bacteria;
- The fitness of an individual in the space of observable Landscapes of Incomplete Fitness Traits (addressed by life-history evolution theory, e.g. balance survival and fecundity to optimize fitness)
- The mean fitness of a population in the space of fitness values of all individuals in the population (by specifiying additional genotype or phenotype information this plane can be collapsed into genotype frequencies or phenotypic traits, producing more traditional views of the adaptive landscape, e.g. mean fitness of a population depending on the frequency of a particular allele)
These levels facilitate connecting biological data from very different disciplines to computer models that help to navigate adaptive landscapes. This opens new avenues for understanding such key topics as robustness, distributions of mutational effects, epistasis, compensatory mutations and adaptive mutations.
Current systems biology models play a pivotal role in this integrated approach, as they have the potential to connect results from molecular biology to higher order emergent properties that might again be observed in experiments. Methods of interest for this approach include the simulation of biochemical reaction networks (Gillespie 2007) and Flux Balance Analyses (Ibarra et al. 2002; Papp et al. 2004).
Numerous practical applications exist for this approach, from cancer research over investigating antibiotics resistance evolution to modelling the consequences of releasing genetically modified organisms (see Loewe 2009 for more examples).
EvoSysBio is necessarily very broad, as it relies on the inclusion of a wide array of research results from very different disciplines. It is not possible to start the integrative work of EvoSysBio without first laying the ground in more specialised disciplines. It is probably also not possible to complete the integrative work of EvoSysBio for any system without going back to the more specialised disciplines to fill in the gaps in our basic knowledge that only become apparent when working on integrated models. Thus successful EvoSysBio is ultimately inclusive, as it strongly depends on the work in many other disciplines.
For a more comprehensive overview of this approach to EvoSysBio, see the most recent
definition of mechanistic EvoSysBio given elsewhere (Loewe, 2016).
References:
Dean, A. M. & Thornton, J. W. (2007) Mechanistic approaches to the study of evolution: the functional synthesis. Nat. Rev. Genet. 8, 675-88. PubMed
Gillespie, D. T. (2007) Stochastic simulation of chemical kinetics. Annu Rev Phys Chem 58, 35-55. PubMed
Ibarra, R. U., Edwards, J. S., & Palsson, B. O. (2002) Escherichia coli K-12 undergoes adaptive evolution to achieve in silico predicted optimal growth. Nature 420, 186-9. PubMed
Lande, R. (2008) Adaptive topography of fluctuating selection in a Mendelian population. J. Evol. Biol. 21, 1096-1105. PubMed
Loewe L (2009) A framework for evolutionary systems biology. BMC Systems Biology 3:27. Journal link
Loewe, Laurence (2015): EvoSysBio in 10 Slides. Figshare 1427128 http://dx.doi.org/10.6084/m9.figshare.1427128
Loewe (2016) Systems in Evolutionary Systems Biology, pp 297–318, vol 4 in: Kliman (ed) Encyclopedia of Evolutionary Biology, Academic Press, Oxford, UK.
Papp, B., Pal, C., & Hurst, L. D. (2004) Metabolic network analysis of the causes and evolution of enzyme dispensability in yeast. Nature 429, 661-4. PubMed
|